Enhancing Blast Efficiency with Predictive Fragmentation Analysis
Introduction
In the field of mining, achieving the best possible fragmentation through precise blasting is incredibly important for efficiency and cost-effectiveness. The Kuz-Ram model is a predictive tool that can forecast fragmentation outcomes and offer strategic advantages in blast planning. In this article, we will explore how the Kuz-Ram model’s predictive capabilities work and its practical application.
Rock Fragmentation
In grasping a fragmentation model, understanding the rock fragmentation process is key. A rock under pressure is more resistant to compression than tension (Persson, Holmberg, & Lee, 1994).
Fragmentation begins when the compressive strength from an explosive shock wave exceeds the rock’s resistance, resulting in pulverization within the borehole hydrodynamic and plastic zone.
Conversely, if the compressive strength is lower, lateral deformation generates fractures or weak cracks (borehole semi-plastic zone). The interplay of compressive and tensile waves, coupled with the gas expansion, governs the material’s fragmentation and movement (Sanchidrián & Muñiz, 2000).
Kuz-Ram Model
As humans have sought to predict the future, mining engineers similarly forecast blast results. One pivotal model in fragmentation prediction is Cunningham’s Kuz-Ram model (2005). It is one of the most favoured methods because excels in the simplicity of data input and directly relates blast design to rock-breaking outcomes (Cunningham, 2005). This model is based on three main equations:
Kuznetsov determines the blast fragment’s mean particle size based on explosives quantities, blasted volumes, explosive strength, and a Rock Factor.
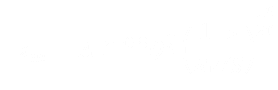
Where: 𝑋𝑚= Medium size of fragments (cm); A = Rock factor; K = Powder factor (kg/m3); Q = Explosive per hole (kg); 115 = Relative Weight Strength (RWS) of TNT compared to ANFO; 𝑅𝑊𝑆 = Relative Weight Strength (RWS) of the used explosive compared to ANFO.
Rosin-Ramler , represents the size distributions of fragmented rock. It is precise in representing particles between 10 mm/0,39 in and 1000 mm/39,37 in (Catasús, 2004, p. 80).
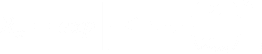
Where: 𝑃 = Mass fraction passed on a screen opening x, n = Uniformity Index.
Uniformity index determines a constant that represents the uniformity of blasted fragments based on the design parameters.

Where: B = burden (m); S = spacing (m); d = hole diameter (mm); W = standard deviation of drilling precision (m); L = charge length (m); BCL = bottom charge length (m); CCL = column charge length (m); H = bench height (m).
The Fusion: O-PitSurface and Kuz-Ram
O-PitSurface seamlessly integrates the Kuz-Ram model, enhancing its blast efficiency capabilities. By synergizing advanced technology with predictive insights, O-Pitblast empowers miners to craft blast designs with unparalleled precision, aligning with specific fragmentation goals.
The recommended method for controlling rock fragmentation is to plan and design the process, check fragmentation predictions, perform the blast, and examine the resulting fragmentation using photo analysis. We suggest using Wipfrag from Wipware for this step. Once completed, the rock factor can be calibrated on O-PitSurface, as demonstrated in the following pictures:
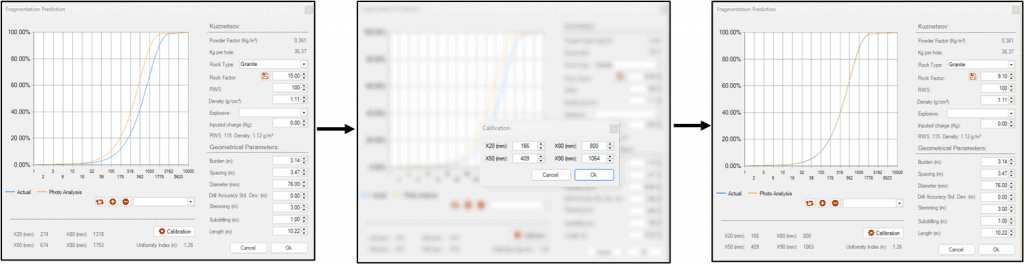
Executing the process multiple times is crucial to boost the accuracy of the fragmentation curve. Ultimately, the Kuz-Ram model plays a vital role in streamlining the drilling and blasting process, which leads to significant cost savings and reduced environmental impact. With precise predictions, proactive blast planning becomes possible, thus ensuring successful operations.
I trust this information will be useful in your daily work!
See you all soon!